How PayJoy Drives Data Trust with Monte Carlo
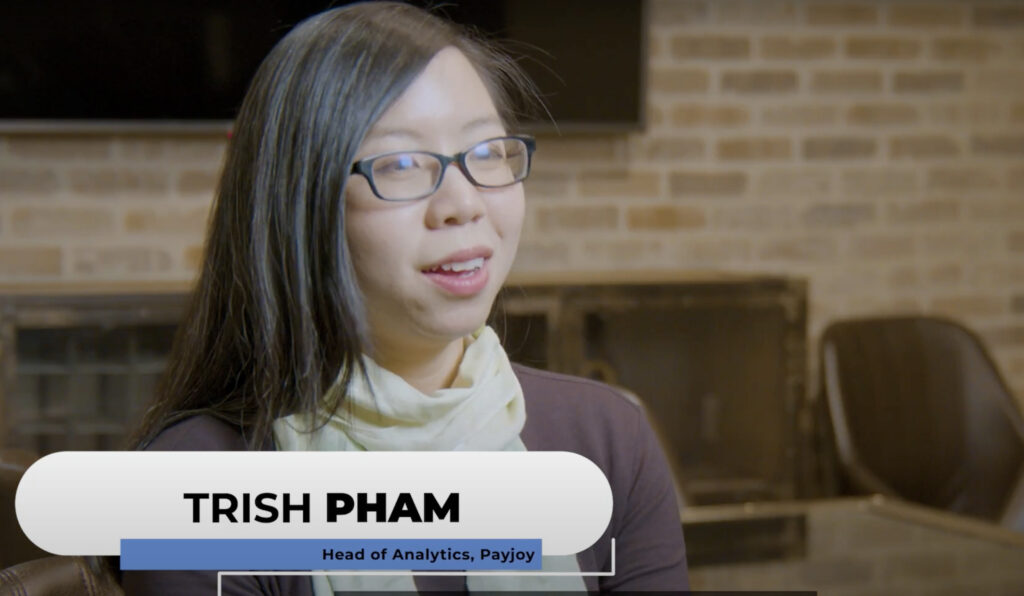
PayJoy is on a mission to bring credit to the next billion people in emerging markets around the world. Using their own proprietary mobile security technology, PayJoy gives customers the ability to afford their first smartphone on credit.
With millions of customers across a dozen countries and support from major industry partners, data reliability plays a key role in PayJoy’s ability to not only deliver on their brand’s promise but also understand their performance and make mission-critical decisions across functions.
Watch the video below or read on to find out how Head of Analytics Trish Pham and the rest of the data team at PayJoy are leveraging data observability to drive data reliability at scale, and discover what’s next on their data roadmap.
PayJoy’s challenge: increasing visibility into data health at scale
PayJoy is a company with a big mission, and they have big data needs to match. With over 2,000 tables, PayJoy uses data to increase their visibility into business performance and enable data-driven decisions across functions.
“PayJoy uses data all across our business groups,” said Trish. “Go-to-market team, our risk strategy team, marketing team…like basically every group that you can think of!”
But with thousands of tables and groups across the organization relying on the quality of their data to make decisions, Trish and her team needed a way to understand data health at scale.
Data observability at PayJoy
To understand the quality of their data and improve data trust across teams, Trish’s team turned to data observability through Monte Carlo.
“Monte Carlo is a very good way for us to understand our data quality at scale,” said Trish.
By utilizing Monte Carlo’s freshness and volume monitors, PayJoy was able to begin monitoring all 2,000 tables in minutes—right out of the box. And by leveraging automated lineage, the team was even able to decrease their time to resolution.
“My favorite feature by far, I would say, is the lineage feature,” Trish went on to share. “It’s also something that whenever I show other people, [they’re] super impressed. They’d be like, ‘Oh, you can see that far?’ Both our Mode reports, as well as the source tables that we’re using to build.”
Leveraging automated lineage into PayJoy’s dbt models for example, Trish’s team is able to immediately understand the downstream effects of a break and root cause the problem before cross-functional data users are ever impacted.
With data observability at the wheel, the data team has not only gained a closer look into PayJoy’s data health—they’ve also improved transparency and data trust across the organization.
So, what’s next for data at PayJoy?
“We’re super excited about the dbt metrics layer. That’s, I think, another step that we’re taking further down the road in terms of not just building the data models themselves but actually defining, like, “Hey, what does sales mean?” Or what do highest level KPIs mean for us” Trish said.
Trish and her team see the metrics layer as a move toward helping cross-functional business users become more self-serve with their data. They hope that by defining critical metrics and their calculations, they’ll be able to alleviate the concerns that often accompany self-service data functions.
“We can verify directly, like, ‘Hey, this is actually a sales metric that we’ve defined in the analytics team. You can feel safe to use us.”
Whatever the future holds, as PayJoy continues to scale their mission-driven organization, their commitment to reliable data adoption—and the infrastructure that powers it—will no doubt play a key role in their success.
Interested in a demo? Schedule a time to see data observability in action:
Our promise: we will show you the product.