Intrinsic Data Quality: 6 Tips Every Data Engineer Needs to Know
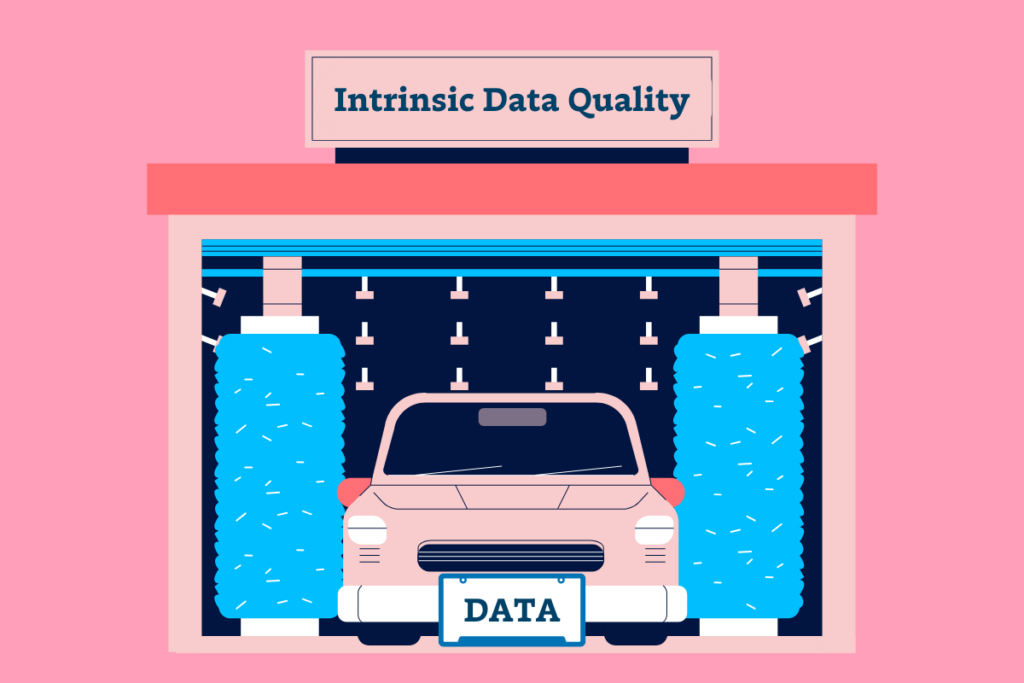
What happens when you strip away all the noise of queries and pipelines and focus on the data itself?
You get down to the intrinsic data quality.
What’s the difference between intrinsic and extrinsic data quality? Intrinsic data quality is the quality of data assessed independently of its use case. Extrinsic data, meanwhile, is more about the context — it’s how your data interacts with the world outside and how it fits into the larger picture of your project or organization.
Consider a database that holds customer details. The intrinsic data quality might involve accuracy, completeness, consistency, freshness, and security. The extrinsic qualities might involve relevancy, reliability, timeliness, usability, and validity.
For example, “Are all the required fields filled in for each customer record?” (completeness) would be a question about the intrinsic quality of the data. On the other hand, “Can the marketing team easily segment the customer data for targeted communications?” (usability) would be about extrinsic data quality.
Each of these aspects is important for maintaining high-quality data that can be used effectively for decision-making, but if you start with high intrinsic data quality, you’re in a better position to address extrinsic quality dimensions effectively.
After all, it’s easier to tailor good data to fit a specific use case than to fix bad data in the first place.
In this article, we present six intrinsic data quality techniques that serve as both compass and map in the quest to refine the inner beauty of your data.
Table of Contents
1. Data Profiling
Data profiling is getting to know your data, warts and quirks and secrets and all. You’re conducting an exploratory analysis of your dataset to understand its general characteristics and identify potential issues. Through data profiling, you can uncover your data’s underlying patterns, relationships, and anomalies.
Once you know what issues are present in your intrinsic data quality, you take steps to address them. You can think of data profiling as the diagnosis and the next technique, data cleansing, as the treatment.
2. Data Cleansing
In the world of data, cleanliness is not just a virtue — it’s an imperative. Data cleansing, sometimes referred to as data scrubbing, involves identifying and correcting or removing inaccuracies and inconsistencies in datasets. Think of it as the digital equivalent of sweeping the floors, dusting the shelves, and clearing out the cobwebs. Except, in this case, the dirt and dust are inaccurate entries, the cobwebs are inconsistencies, and the room is your database.
The process of data cleansing can involve various tasks like removing duplicates, correcting spelling errors, filling in missing values, and standardizing data formats. The aim is to ensure that the intrinsic data quality is high enough that the data is accurate, complete, consistent, and ready for use.
3. Data Validation
Data validation involves checking data against certain rules or standards before it’s used or entered into your database. It’s about guaranteeing that the data is accurate, consistent, and suitable for your needs — a critical step in maintaining the intrinsic data quality of your datasets.
Data validation can take many forms, depending on the nature of your data and your specific requirements. For example, you might validate that email addresses follow the correct format, that date fields contain valid dates, or that mandatory fields aren’t left blank. You might also check that numerical entries fall within a certain range, or that text entries don’t contain illegal characters. All of these data validation practices help to guarantee that your intrinsic data quality is accurate and fresh.
4. Data Auditing
Why is regular data auditing so important? Imagine you’re running a business. You wouldn’t wait until your finances are in disarray before checking your accounts, would you? Similarly, you shouldn’t wait until your data is causing problems before checking its quality. Regular data auditing allows you to catch and correct issues before they potentially escalate into larger problems.
By regularly reviewing your data, you can gain insights into trends, patterns, and potential areas for improvement. You might discover, for example, that a particular data source is consistently producing errors, indicating a need for better data collection methods. Or you might find that a certain type of data is particularly valuable for your analyses, suggesting a focus area for future data collection efforts. Monitoring your data consistently, especially through the use of a data observability tool, is an effective way to ensure your data is consistently fresh, accurate, and up-to-date by automatically alerting your team to potential discrepancies.
5. Data Governance
Next up is creating a clear roadmap for your data management efforts, outlining the processes to follow, the roles to play, and the responsibilities to uphold. This is known as data governance.
Without defined processes, managing your data could become a haphazard effort. Designated roles over who’s responsible for what across your data management workflows is essential to building intrinsic data quality. Without established responsibilities, there might be a lack of accountability for data quality, potentially leading to errors and inaccuracies. Data governance brings order to this potential chaos by defining who can take what action, upon what data, in what situations, using what methods.
6. Use of Data Quality Tools
Let’s face it: there are a lot of manual tasks involved in maintaining intrinsic data quality. Data quality tools, however, can free up your team to focus on higher value tasks that can’t be automated.
A data observability platform, like Monte Carlo’s, can help. It employs machine learning to empower data engineering teams to resolve data issues more rapidly. Once linked to your data environment through no-code onboarding, it can identify data issues, assess impact, and send alerts. Teams can then trace the root cause faster, reducing the time spent on data fire drills and downtime.
The platform takes about a week to learn the data environment and benchmark, after which it’s ready to enhance your intrinsic data quality.
Refresh your intrinsic data quality with data observability
Ready to simplify your process to maintain high intrinsic data quality and reliable data pipelines? Monte Carlo’s data observability platform is here for you. Chat with our team to see why it’s easier than ever to refine your data’s inner beauty, turning raw, rough data into the polished gems that can drive your business forward.
Our promise: we will show you the product.