Monte Carlo Brings Data Observability to Data Lakes with New Databricks Integration

As companies leverage more and more data to drive decision-making and maintain their competitive edge, it’s crucial that this data is accurate and reliable. With the new Databricks integration from Monte Carlo, teams working in data lakes can finally trust their data through end-to-end data observability and automated lineage of their entire data ecosystem.
Over the last few years, data lakes have emerged as a must-have for the modern data stack. They often offer more flexibility and customization than traditional data warehouses, but data engineers know there’s usually a tradeoff when it comes to data organization and governance. And those issues can be costly: research from the last few years suggests that companies are losing millions of dollars in wasted revenue while data teams are wasting nearly 50% of their time fixing broken pipelines and other data quality issues.
At the heart of this problem is data downtime, those occasions when data is missing, stale, incomplete, or otherwise inaccurate. And it’s why we’re excited to announce that we’re bringing data observability—the solution to data downtime—to data lakes with the new Databricks integration for Monte Carlo.
What is data observability?
Inspired by the proven best practices of application observability in DevOps, data observability is an organization’s ability to fully understand the health of the data in their system. Data observability, just like its DevOps counterpart, uses automated monitoring, alerting, and triaging to identify and evaluate data quality issues.
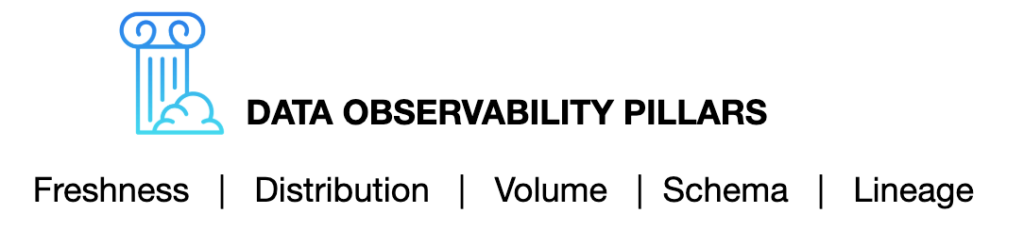
At Monte Carlo, we look at data observability across five pillars:
- Freshness—how up-to-date your data tables are
- Distribution—whether your data falls into expected, acceptable ranges
- Volume—the completeness of your data tables
- Schema—changes in the organization of your data
- Lineage—the upstream sources, downstream ingestors, and interactions with your data across its entire lifecycle
Our data observability platform uses machine learning to infer and learn what an organization’s data looks like in order to proactively identify data downtime, assess its impact, notify those who are responsible for fixing it, and enable faster root cause analysis and resolution.
The unique challenge of observability in data lakes
For teams that use Databricks to manage their data lakes and run ETL and analysis, data quality issues prove especially challenging. Data lakes almost always contain larger data sets, often with massive amounts of unstructured data. They also usually require many components and technologies that need to work together, opening up more potential opportunities for pipelines to break. And while data engineers working in other tech stacks can leverage data testing tools like dbt and Great Expectations, scaling these solutions for the large datasets typical of data lakes can prove challenging.
The consequences of data quality issues in data lakes can be significant, especially when it comes to machine learning. ML is a huge application for data lakes, but if the data feeding those models isn’t accurate and trusted, the output will be compromised. As ML leader Andrew Ng recently said, “The full cycle of a machine learning project is not just modeling. It is finding the right data, deploying it, monitoring it, feeding data back [into the model], showing safety—doing all the things that need to be done [for a model] to be deployed. [That goes] beyond doing well on the test set, which fortunately or unfortunately is what we in machine learning are great at.”
Ensuring data quality is paramount for any ML practitioner—and really, for any data-driven organization. And now, with the Databricks integration from Monte Carlo, reducing or even eliminating data downtime across a data lake is possible.
How the Monte Carlo’s Databricks integration works
Our new integration makes it possible for data teams working in Databricks to layer automated monitoring and alerting on top of their data tech stack, including within their data lakes. Our integration is designed to easily scale to environments with hundreds of thousands of tables, and datasets of any size. Monte Carlo also provides automated, scalable data lineage, delivering a holistic map of an organization’s data across its entire lifecycle that teams can use to quickly identify and address root causes and potential impacts of data downtime. And with Monte Carlo’s SOC-2 certification, Databricks customers can rest assured that their data is kept secure and all best practices will be met.
As Databricks co-founder Matei Zaharia told us recently, “AI and machine learning really should have been called something like ‘data extrapolation’, because that’s basically what machine learning algorithms do by definition: generalize from known data in some way, very often using some kind of statistical model. So when you put it that way, then I think it becomes very clear that the data you put in is the most important element.”
We couldn’t be more excited to see what’s in store for the future of data lakes. And with our new Databricks integration, the data powering this future just became a lot more reliable and trustworthy.
Ready to achieve end-to-end data observability that encompasses your data lake? Reach out to Itay Bleier to learn more about how Monte Carlo and Databricks work together.
Our promise: we will show you the product.