Monte Carlo’s Series D and the Future of Data Observability
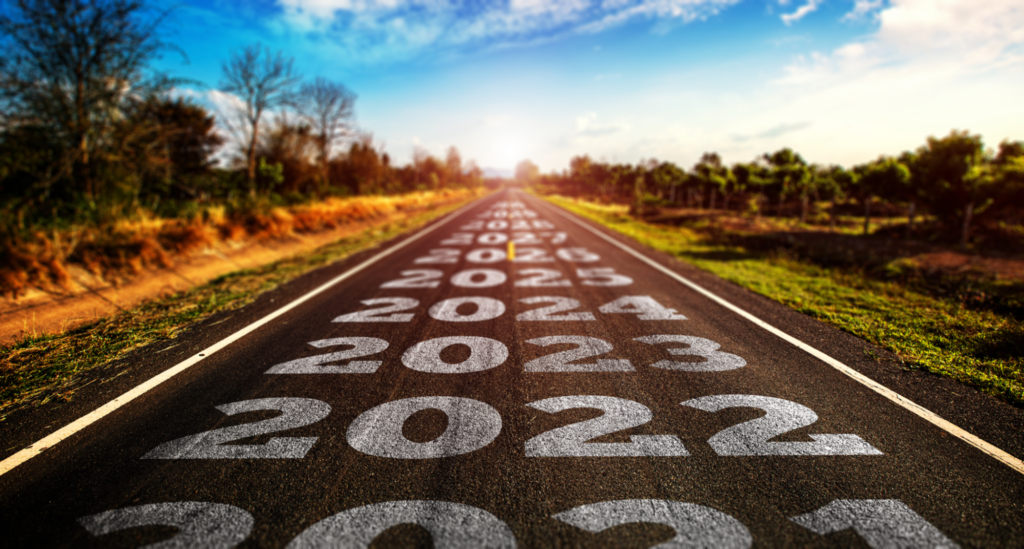
Four years ago, I was leading a data team at Gainsight, a customer success company, responsible for generating the analytics that powered our executive dashboards. I was regularly getting questions and pings from downstream consumers like “This data is wrong.”
It was a painful experience, but unfortunately, it wasn’t unique. As it turns out, I wasn’t the only one who felt this pain.
Before launching Monte Carlo, I spoke with hundreds of data leaders who all struggled to deliver high quality data despite having their teams spend upwards of 30% of their time and millions of dollars per year on this issue.
And the problem is only getting bigger. Just a few weeks ago, Unity, the popular gaming software company, cited “bad data” (or as it’s often called, data downtime) for a $110M impact on their ads business.
Since we launched in 2020, Monte Carlo has helped hundreds of customers tackle broken data pipelines, stale dashboards, and other symptoms of poor data quality with data observability.
With our Series D, our goal is to make our customers as happy as possible by accelerating the data observability category and eliminating data downtime. Here’s how we got here and where we’re going next.
Where data observability is today
Organizations spent $39.2 billion on cloud databases such as Snowflake, Databricks, and BigQuery in 2021. Unfortunately, those investments have not been fully realized with poor data quality costing the average organization $12.9M per year.
Data observability fundamentally changes this equation. It’s amazing to see the value innovative data teams like Vimeo, Autotrader, PagerDuty, and others can provide when their organizations trust their data. For example:
- Fox empowered self-service across a decentralized data organization.
- Red Ventures improved data delivery by tracking performance via SLAs.
- Blinkist grew revenue by providing their marketing team with data to better optimize ad spend.
- JetBlue accelerated their data quality processes to match the real-time needs of their business.
- PagerDuty protected their customer success machine learning models from drift.
- Choozle made high-quality data a differentiating factor in their updated digital advertising platform.
- Clearcover scaled a lean data team, removed bottlenecks, and increased data adoption.
- ShopRunner enhanced visibility data asset usage allowing them to optimize their environment without impacting downstream users.
- Hotjar reduced their data infrastructure costs 3x.
All of these organizations also dramatically reduced data downtime so their engineers could spend more time innovating and less time fixing.
Industry observers have taken note. Gartner is providing guidance on data observability and it’s cited by data teams on G2 as a “game changer” for the modern data stack.
This is deeply validating for those passionate about data reliability, but the best is yet to come.
The future of data observability and Monte Carlo
The markets for data broadly continue to signal an incredible opportunity for data observability. Last year, BigQuery, Snowflake, and Databricks annual revenues each neared or exceeded $1b, and in 2021, they continue to grow at astounding rates.
We hear often from data teams and industry analysts that data quality has or is becoming a top priority, as companies look to capitalize on data infrastructure investments to drive growth and maintain their competitive edge.
When it comes to data observability specifically, the trajectory of site reliability engineering and application performance monitoring (APM) may provide a glimpse of what’s to come.
Datadog, a leading application performance monitoring (APM) solution, is becoming one of the most iconic companies of the decade, with over $1b in revenue in 2021. The idea of spinning up an application without some form of APM or observability to ensure uptime and minimize downtime is practically unheard of.
With this raise, Monte Carlo remains focused on making our customers as happy as possible.
This means building an incredible, end-to-end data observability product with more integrations, more detailed lineage, enhanced support for data lakes, deeper insights into data health, and best-in-class alerting to reduce time to detection and resolution for data incidents.
It also means listening and engaging with the data community to develop and advocate for a set of data quality best practices.
To that end, we are pleased to be publishing “Data Quality Fundamentals” with O’Reilly Media later this fall, and sponsoring Snowflake Summit next month. (We’ll be there – come say hi!)
As a data leader, what if frantic Slack messages about missing values or early morning fire drills when pipelines break were a thing of the past?
What if trusting your data was as easy as creating a new dashboard in Looker or Tableau, or spinning up analytic workloads in Snowflake or BigQuery?
What if you could prevent data quality issues – and downstream before they even happened?
Think: end-to-end coverage across your entire stack, no matter what tools you use; SLAs, SLIs, and SLOs to manage data availability, freshness, and performance; a centralized control panel to help you troubleshoot data issues immediately while giving you a 10,000-foot view of data health over time. The possibilities for better data are endless.
By executing on this vision and focusing on what matters (you – our customers!), we can eliminate data downtime and bring reliable data to companies everywhere.
Book a time to speak with us in the form below.
Our promise: we will show you the product.