Stale Data Explained: Why It Kills Data-Driven Organizations
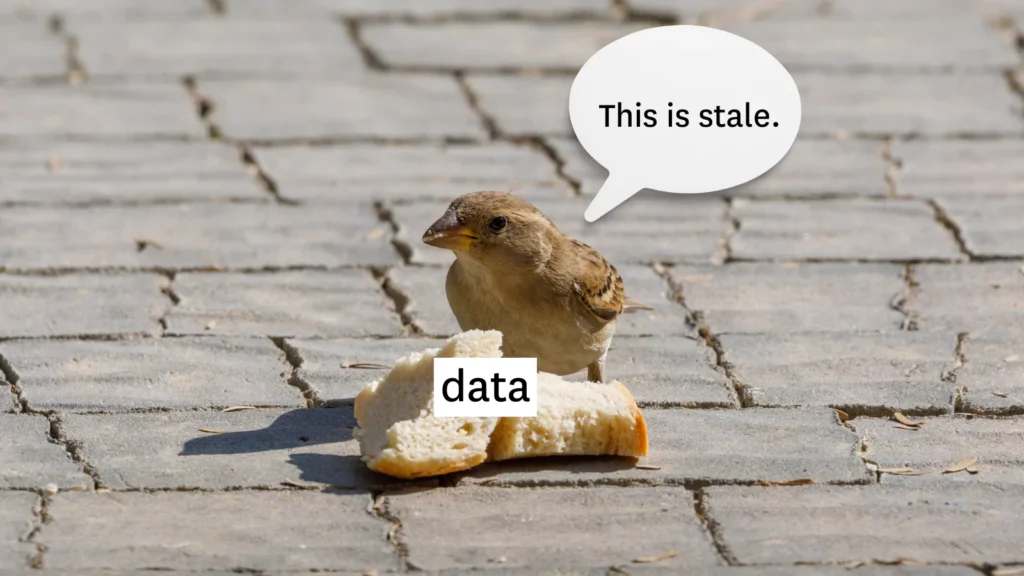
What is stale data?
Stale data is data that hasn’t been updated at the frequency interval required for its productive use. Depending on the use case, this could be weeks or minutes. It’s completely contextual.
For example, the marketing team may require their ad spend dashboard updated weekly for their regular meeting where they make optimization decisions, but a machine learning algorithm that detects financial fraud may require data latency measured in seconds (or less).
As Randy Addis, head of data and business intelligence at Best Egg puts it:
“Now, it’s not necessarily worrying about how we are going to get the data from server A to server B, because it’s all connected in the cloud. Now it’s more about we have such a breadth of data—how do we manage it and make it more easily discoverable and more easily searchable? How do we instill the sense of quality and timeliness in the data?”
How does stale data happen and what are the consequences?
Stale data can be the result of various factors, such as outdated data collection processes, bad SQL queries, and broken data pipelines.
It is a data quality issue also known as data completeness, freshness, or timeliness. But whatever it’s called, the consequences are potentially severe. It doesn’t matter if the data is accurate if it arrives too late to take action.
Stale data can undermine an organization’s trust in data or lead to inaccurate insights, misguided decisions, and missed opportunities. In today’s fast-paced business environment, that creates organizational inefficiencies that hinder growth.
For example, we know of one e-commerce company that lost millions of dollars due to stale data, which prevented their out-of-stock recommendation machine learning model from working as intended. On the other hand, fresh data can help improve the accuracy and effectiveness of machine learning models, leading to more accurate predictions and better business outcomes.
Stale data can also lead to wasted data engineering hours and increased labor costs. Instead of building valuable pipelines or further extracting value from data, engineers are running around fighting data quality fire drills. In fact, our survey shows that data professionals spend an average of 40% on data quality related tasks and our internal product telemetry suggests data freshness accounts for nearly ⅓ of data downtime related issues.
How is it measured?
Stale data is best measured like any other data reliability metric. You measure the amount of time the data is updated on time and divide by the amount of time it is stale.
This can be done on the aggregate level across your data warehouse or by domain or even by data product (or production table!). Many data teams such as Red Ventures, choose to develop custom service level agreements or data SLAs.
This allows the data team to better understand the requirements of their data consumers and provide transparency into the uptime those consumers can expect from their data products.
This helps establish data trust and allows data leaders to better prioritize where to spend additional data engineering time and resources to improve data quality and prevent stale data.
How do you prevent stale data
The scale of data and the number of integration points across today’s modern data stack are immense.
It is virtually impossible to expect any human (or manually defined data tests) to be able to detect every instance of stale data across the organization. And once detected, it is even harder to trace the root cause of data incidents to one of the near infinite number of possibilities.
This is why leading data teams like Vimeo, Fox, and others have started to leverage data observability solutions that leverage machine learning to automatically detect and accelerate the resolution of data incidents. Stale data (data freshness) is one of the five pillars of data observability.
Leveraging these solutions can reduce data downtime by 80 percent or more.
Don’t let data get stale
Stale data is a significant threat to the reliability and usefulness of data. Stale data can erode user trust in data, undermine the accuracy of machine learning models, and hinder the development of valuable data products.
By maintaining data freshness through automated processes, data quality tools, and clear data governance strategies, businesses can ensure that their data is reliable and trusted.
Interested in how data observability can help you better detect and resolve stale data before it becomes a problem? Fill out the form below to schedule a time to speak with us!
Our promise: we will show you the product.