The Cost of Bad Data
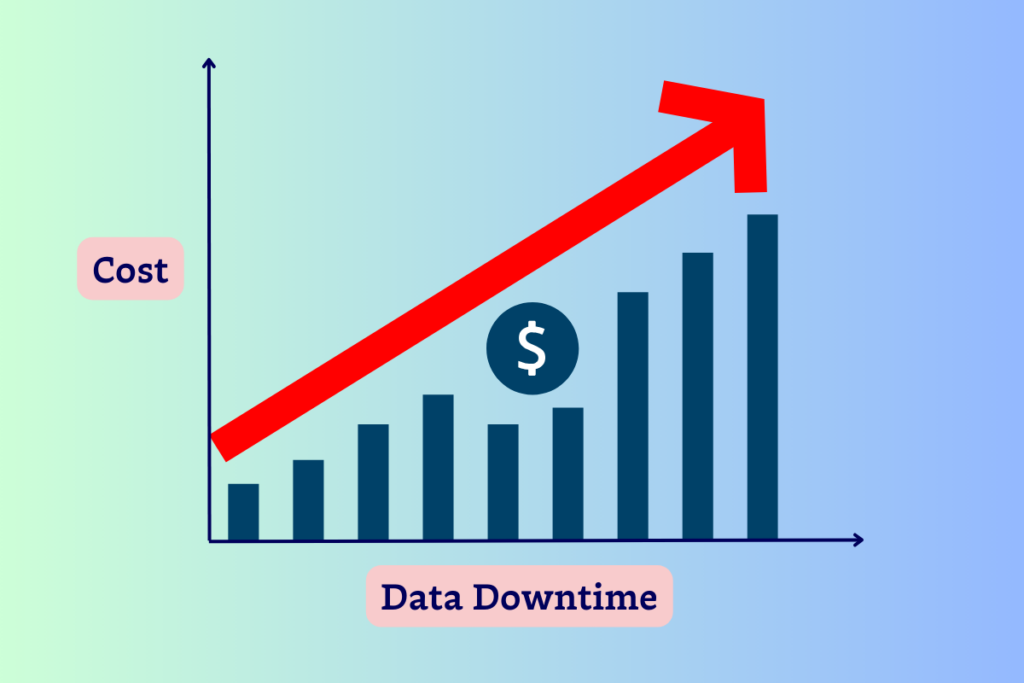
When data engineers tell scary stories around a campfire, it’s usually a cautionary tale about bad data.
Data downtime can occur suddenly at any time—and often not when or where you’re looking for it. And its cost is the scariest part of all.
But just how much can data downtime actually cost your business? In this article, we’ll learn from a real-life data downtime horror story to understand the cost of bad data, its impacts, and how to prevent it.
Table of Contents
First, how do you calculate data downtime?
Data downtime—as we’ve come to call it—is the period of time when your data is partial, erroneous, missing, or otherwise inaccurate. Data downtime can be calculated with a relatively simple equation:
DDT = N x (TDD + TTR)
or
Data Downtime = (Number of incidents) x (Average time to detection + Average time to resolution)
So, now let’s define each of the variables in a bit more detail:
- DDT: periods of time when data is erroneous, missing, or inaccurate
- N: the number of instances of incorrect, missing, or incomplete data over a given time period
- TTD: the average time from when incidents occur to when they are detected
- TTR: the average time from when the issue has been detected to when it has been given a resolved status
Our estimates suggest data teams spend 30–40% of their time handling data quality issues instead of working on revenue-generating activities.
To give an idea of just how costly data downtime can be for an organization, a $50m company with 3,000 tables, a team of 5 data engineers, and medium data dependence, suffers from 793 hours of data downtime a month on average.
Yes – you read that number right.
According to the data quality calculator, that 793 hours would cost an organization roughly $195,734 in resource costs to fix the issues and $683,931.51 in inefficient operations during data downtime periods.
One CDO even reported that his 500-person team spends an astounding 1,200 cumulative hours per week tackling data quality issues.
When bad data strikes, it means (bad) business. Sometimes the financial consequences are salvageable. In extreme cases, they can take a business down completely. Let’s consider the latter.
The (worst case) cost of bad data
At a recent data leader’s panel we hosted, we discussed a real data downtime scenario that led to a business actually closing its doors for good.
“At a freight tech marketplace, the data team had crafted a valuable machine learning model that predicted the auction ceiling and floor of truckers’ possible bids for particular shipments. The model worked by training on all the data that was happening in the marketplace, and helped the company know whether it was profitable to put a given shipment on the market or not.
But another team within their organization had introduced a new feature that automated that bidding process for the drivers — improving their experience, but unknowingly infecting the valuable auction model by routing that “autobid” data back for training. As the data team discovered too late, the model no longer predicted the actual behavior in the marketplace, but rather, only predicted the company’s own automation.”
A scary story, indeed. A simple coding or schema change can wreak havoc on an organization.
Let’s say this company’s annual revenue was $65M, and they leveraged 6,000 tables for the ML model alone, a team of 4 ML data engineers, and a high data dependence. Our calculator estimates the cost of this bad data would be:
- 400 data incidents per year
- 2400 data downtime hours per year
- $156,587 in resource cost
- $2,671,232 in efficiency cost
These numbers are, of course, hypothetical, but they’re significant. They represent the massive financial consequences of a data discrepancy.
In reality, they may not even be too far off – the corruption in training data for this model led to millions of dollars in lost revenue and the company has since shuttered.
Now, let’s take a look at a few other data downtime scenarios – this time, with quicker resolutions and happier endings.
The cost of bad data: A $500,000 data quality issue
From its humble beginnings as a mobile ringtone provider, omnichannel advertising platform Kargo has experienced dramatic scale since it opened its doors in 2013. After multiple acquisitions and updates to the business, the Kargo team consolidated their data platform in Snowflake to centralize their data function, and they built a data stack consisting of Airflow, Looker, Nexla, and Databricks.
But transforming their data architecture was just one small step. The giant leap came with ensuring the quality of their data at scale.
The team required reliable, consistent, and accurate data to not only provide customer deliverables and campaign reporting, but to support the very ML models that powered Kargo’s media buying operations. Both of these data products are crucial to the success of Kargo’s business – and any downtime in these pipelines would have serious financial consequences. On one occasion, about half a million dollars in consequences.
Kargo’s VP of Analytics, Andy Owens, outlines a data quality issue that arose for the team in 2021: “One of our main pipelines failed because an external partner didn’t feed us the right information to process the data. That was a three day issue that became a $500,000 problem.”
So what was this great data quality issue that caused so much trouble?
Just a common case of inaccurate partner information.
On its surface, the discrepancy seems harmless enough. Minor keying errors happen all the time. But, as every good data engineer knows, any minor discrepancy be it a misplaced 0 or a volume, freshness, or bad schema change—if not caught and resolved quickly—can have ripple effects that wreak havoc on your data products.
“We’ve been growing at double digits every year since then so if that incident happened today [the impact] would be much larger,” said Owens.
A $20,000 data quality crisis averted
For Kargo, data observability was the clear solution to prevent this kind of data quality issue – and it made an immediate impact.
“A developer released something on a Friday afternoon and within three hours reverted the code. I asked them, ‘what happened, how did you know to turn this off?’” said Owens. “He said, ‘I saw transaction volumes tanking [from a data volume alert sent by Monte Carlo] so I turned it off.’”
Had the developer not been alerted to this issue and reverted the code, Owens estimated that “it would have cost Kargo approximately $20,000.”
“Previously, our developers didn’t have visibility or feel ownership of these business problems. They shipped and QAed,” explained Owens “They perform fixes based on alerting now.”
With data observability on its side, Kargo is able to proactively monitor and manage data quality—and provide much needed resolution support for the data teams who own it.
Preventing lasting impacts of bad data at scale with data observability
Good data is valuable. Bad data is expensive.
Bad data isn’t just an inconvenience. Left unchecked, bad data erodes trust, burns out data teams, and wreaks financial havoc on unprepared organizations. But most data teams don’t have the time—or the tools—to adequately manage data quality on their own.
That’s why automated data observability is so important.
Data observability can help manage bad data before it snowballs. With a solution like Monte Carlo, data teams not only get alerted to data quality issues sooner, but they can manage and resolve them faster—with tools like automated data lineage to understand where pipelines broke, what was impacted, and who needs to know about it.
As Owens said, “Data quality can be death by 1,000 cuts. With Monte Carlo we have meaningfully increased our reliability levels in a way that has a real impact on the business.”
Want to learn more about how data observability can stop bad data in its tracks? Give us a call!
Our promise: we will show you the product.